Revolutionizing Climate Modeling: How SFNO Enhances Weather Forecasting on a Global Scale
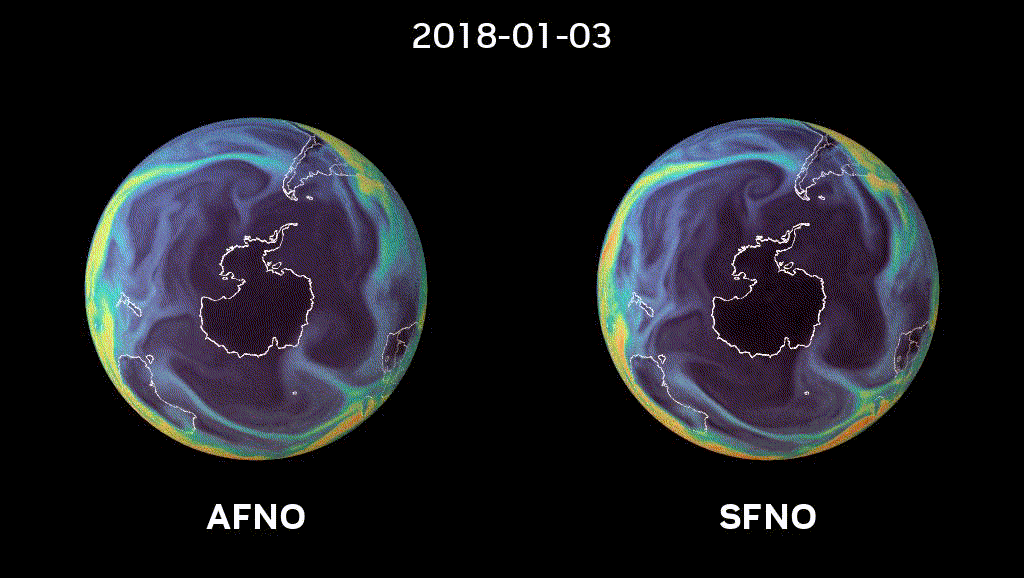
Navigating the Challenges of Weather and Climate Modeling with Fourier Neural Operators
When it comes to navigating the effects of climate change, one of the greatest challenges we face today is modelling Earth's complex weather and climate systems accurately and efficiently. This task is not just a scientific endeavour but a necessity for understanding and adapting to our changing world. Among the various methods employed for this purpose, Fourier Neural Operators (FNO) have emerged as a promising tool. FNOs are known for their ability to handle complex modelling tasks, which is essential in predicting weather patterns and climate changes.
However, a significant hurdle arises when dealing with spherical data, which is integral to global weather and climate modelling. Traditional FNOs, while powerful, aren't inherently designed to handle the spherical geometry of our planet. This limitation poses a critical gap, especially considering the global nature of climate phenomena and the need for accurate modelling across the Earth's entire surface.
Introducing Spherical Fourier Neural Operators: A Leap Forward in Global Weather Forecasting
Recognizing the limitations of traditional Fourier Neural Operators in spherical data handling, a groundbreaking solution has emerged: Spherical Fourier Neural Operators (SFNO). This innovative approach is a game-changer, combining the strengths of FNOs with an enhanced capability to process spherical data. What sets SFNO apart is its alignment with the fundamental expectation that physical laws should remain consistent, regardless of the frame of reference. This principle of rotational equivariance is a cornerstone of SFNO, ensuring that it accurately represents the spherical nature of our planet in climate modelling.
The beauty of SFNO lies in its grid-invariance, allowing models to be re-trained at different configurations and resolutions. This flexibility is crucial for adapting to various climate modelling scenarios, ranging from regional to global scales.
SFNO in Action: Impressive Results and Unmatched Stability
When put to the test, SFNO's capabilities dazzle. Applied to the Earth Reanalysis 5 dataset and the Spherical Shallow Water Equations, SFNO demonstrated remarkable long-term stability in predictions. In contrast to the 25-day stability of FFT-based methods, SFNO maintained its predictive accuracy for over a year. This level of stability, combined with the computational efficiency of processing each step in less than 13 minutes on an NVIDIA A6000 GPU, marks a significant advancement in long-range weather forecasting and uncertainty quantification.
The Future of Climate Modeling: SFNO's Integration into NVIDIA Modulus and Siml.ai
The implications of SFNO's success extend far beyond academic circles. Its integration into the latest version of NVIDIA Modulus is a testament to its practical utility and potential impact on the field of climate modelling. In the near future, the users of Siml.ai can look forward to harnessing the power of SFNOs in their weather and climate predictions, benefiting from their enhanced accuracy and efficiency. This development marks a significant milestone in our quest to understand and adapt to the ever-evolving dynamics of Earth's climate. For further reading, we refer to https://arxiv.org/pdf/2306.03838.pdf.
Credits: Thumbnail .gif was downloaded from the NVIDIA Developer blog "Modeling Earth’s Atmosphere with Spherical Fourier Neural Operators" by Bonev B. et al. (2023)