Aerodynamic Simulation: Transforming with State-of-the-Art Neural Network Architectures
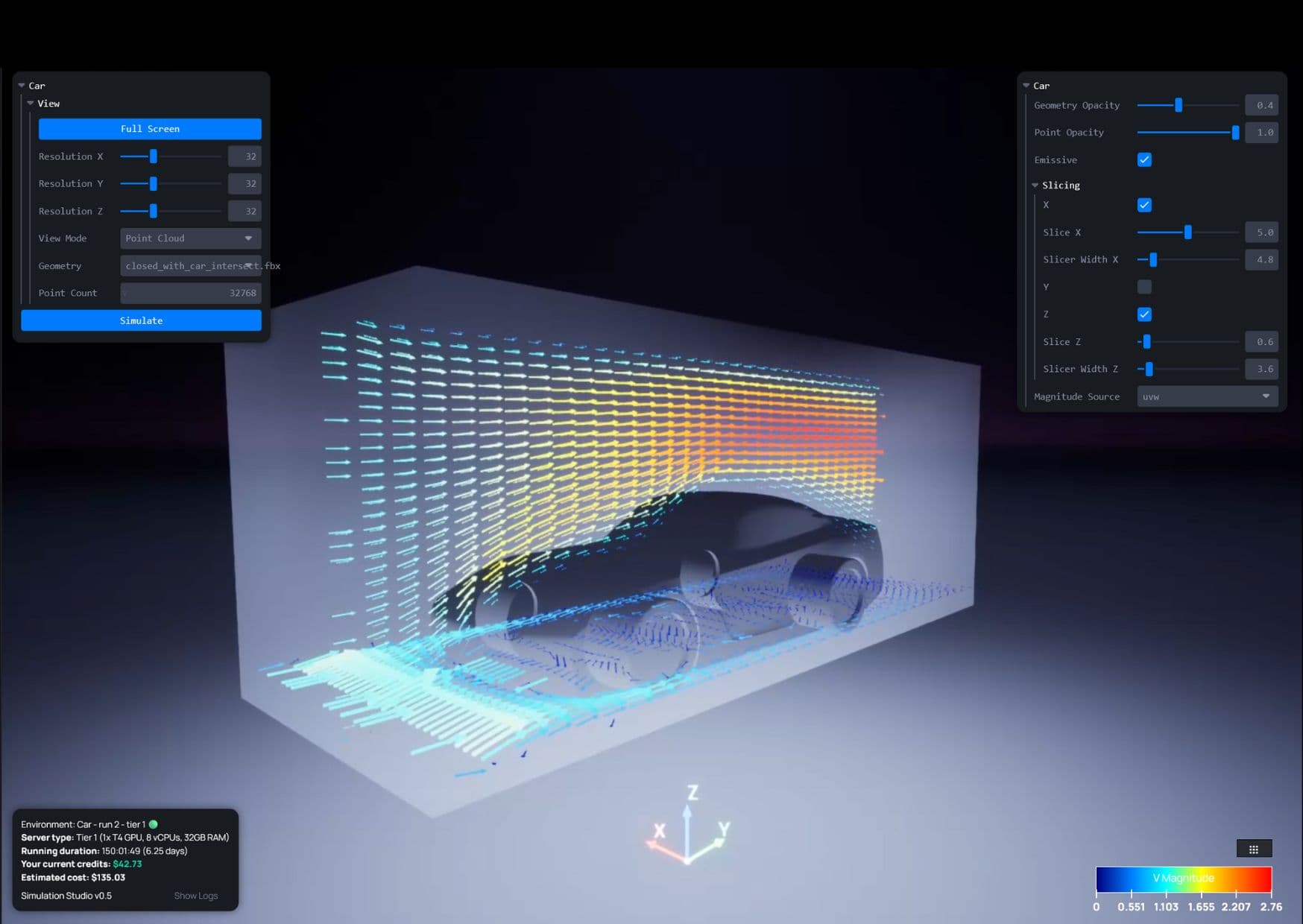
We recently published a research paper titled "Simulation Analysis of Car Aerodynamics within Tunnel Environment using Deep Learning: A Comparative Architectural Study". Presented at The 6th International Conference on Pattern Analysis and Intelligent Systems, the paper will soon be available in the conference proceedings and be included in the IEEE Explorer index.
Introduction
Aerodynamic simulation is a critical aspect of automotive design, which is pivotal in optimizing fuel efficiency, stability, and overall vehicle performance. Traditionally, computational fluid dynamics (CFD) methods and wind tunnel testing have been the go-to tools for analyzing airflow patterns and optimizing vehicle designs. However, these methods often demand substantial computational resources and time, especially when simulating complex fluid-structure interactions under various speeds and conditions. In light of this, recent advancements in machine learning, specifically neural network architectures, have opened new avenues for simulating physical phenomena, including fluid dynamics.
Literature Review
The paper "Simulation Analysis of Car Aerodynamics within Tunnel Environment using Deep Learning: A Comparative Architectural Study" explores the utilization of advanced neural network architectures, specifically Fully Connected Neural Networks (FCNN) and Sinusoidal Representation Networks (SiRen), for simulating aerodynamic flow around a vehicle in a tunnel environment via computational fluid dynamics. The study compares the performance characteristics of these architectures, highlighting their potential to revolutionize automotive design processes.
Methodology
The methodology employed in the study encompasses a detailed examination of the computational setups, simulation parameters, and performance metrics used to assess the effectiveness and efficiency of each architectural configuration. The research compares three distinct computational architectures, characterized by their hardware and software configurations, to understand their impact on simulation outcomes.
Results and Discussion
The results of the study reveal significant insights into the application of FCNN and SiRen Architecture in simulating car aerodynamics within a wind tunnel environment. Through rigorous simulation and analysis, the performance of both neural network models across various airflow conditions is evaluated, focusing on their accuracy, computational efficiency, and visual agreement with traditional Computational Fluid Dynamics (CFD) results.
Conclusion
The study underscores the delicate balance between computational demand and time efficiency in aerodynamic research. By optimizing computational strategies and leveraging suitable neural network architectures, researchers can effectively navigate the complexities of aerodynamic simulations while adhering to budgetary constraints. Moreover, the findings highlight the significance of standardizing computational methodologies and server configurations to ensure reproducibility and efficiency across different analyses.
The study provides valuable insights for enhancing computational aerodynamics methodologies, guiding future research endeavors toward achieving greater precision and efficiency in aerodynamic simulation and analysis. By harnessing the potential of advanced computational tools and methodologies, researchers can continue to push the boundaries of aerodynamic research, driving innovation and advancement in automotive engineering and beyond.
In conclusion, the paper sheds light on the potential of advanced neural network architectures to revolutionize aerodynamic simulation, paving the way for more efficient and accessible simulation methods for automotive design and beyond. The findings of the study offer valuable insights for researchers and practitioners in the field of aerodynamics, highlighting the importance of computational efficiency and accuracy in simulating complex fluid dynamics.